Automotive
AI in the Driver's Seat
Whether deployed in-cabin for driver distraction applications or in the advanced driver-assistance system (ADAS) stack for object recognition and point cloud processing, AI forms the backbone of the future of safer, smarter cars.
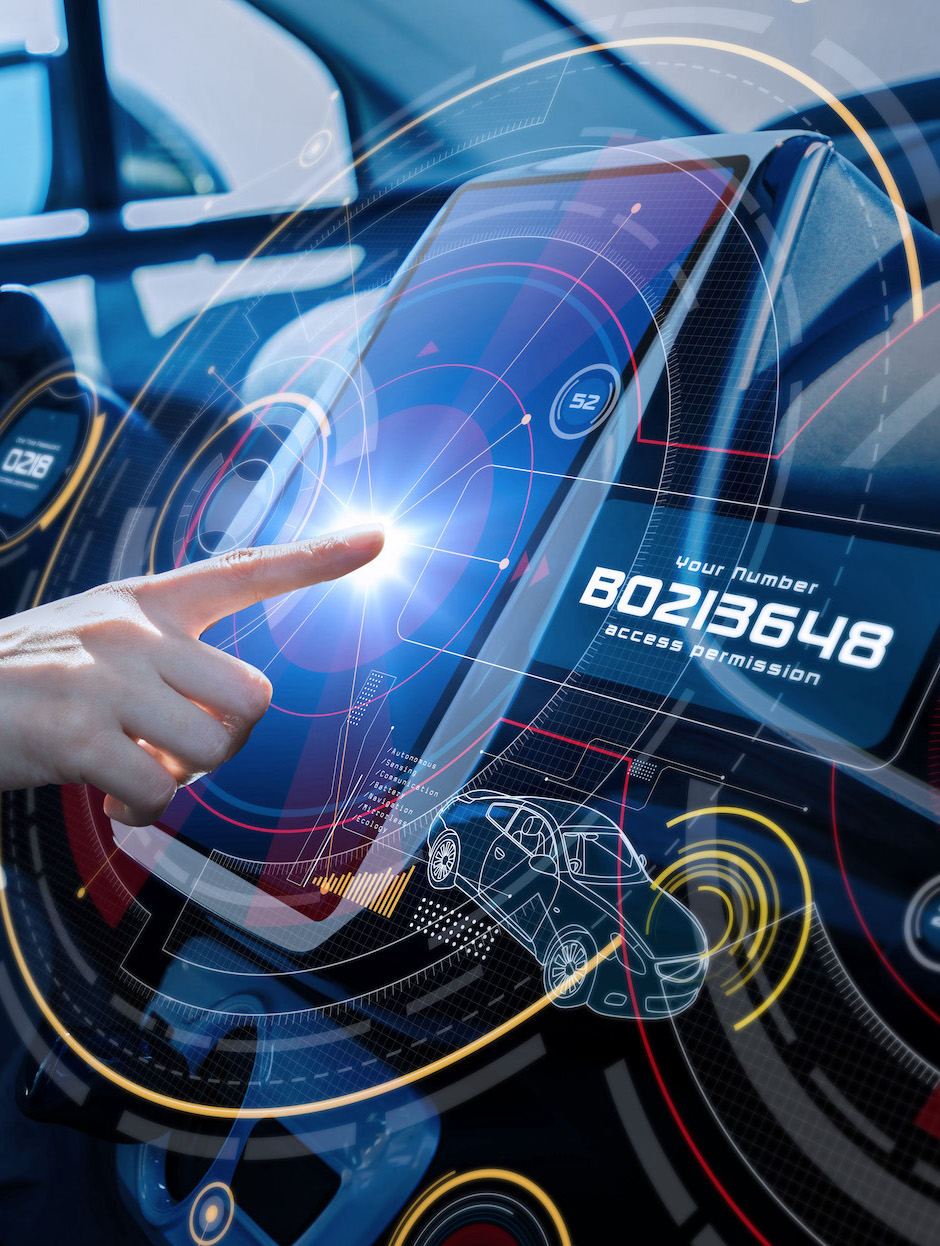
Faster Power-Efficient Processing
It’s still surprising, but not uncommon, to occasionally spot a driverless robotaxi on the streets of San Francisco, Austin, or Phoenix. However, most experts agree that we are still years away from replacing human drivers with autonomous vehicles. Much of the technology that got the industry to Level 3 will not scale in all the needed dimensions—performance, memory usage, interconnect, chip area, and power consumption—to Level 5, where no human intervention is required. The scalability problem is perhaps no better illustrated than in Artificial Intelligence (AI) processing. Automobiles are increasingly data-centric, with AI leading the charge. NPUs (Neural Processing Units) offer a scalable, power-efficient AI processing solution customized for automotive applications.
Scaling Performance – How Many TOPS Do I Need?
The performance required for full self-driving autonomy arguably exceeds today’s capabilities. There has yet to be an industry consensus on how much additional processing capability is needed. For discussion, consider that a self-driving vehicle may need six to eight 8K camera inputs and data from LIDAR, radar, ultrasonic, and other sensors. ADAS will continue to drive increases in TOPS (Trillions of Operations per Second) requirements. Still, it is unclear how additional processing capability will be used. Just a few years ago, some estimated 24 TOPS would be required for L3—a figure that today’s automakers have already surpassed with new estimates from 150 to 200 TOPS. Factors in the exponential processing needs for L4 and L5, and a cursory study of today’s mainstream architectures, show that the industry needs a new AI processing paradigm. Active cooling of chips within cars is neither ideal nor commercially feasible, and the costs of using multiple large, leading-node semiconductors don’t fit most carmakers’ financial models.
Perceiving the World with Sensor Fusion
Autonomous vehicles perceive the world using sensor fusion. They interpret environmental conditions collectively, taking inputs from RADAR, LiDAR, cameras, and ultrasonic sensors and making sense of the bigger picture to deliver the information needed for an autonomous vehicle to operate safely. Sensor fusion processing requires concurrently and efficiently running multiple unique Neural Networks (NN)—something not typically seen in other markets. For example, a small consumer device may run a single NN with a small resolution, such as ResNet50 at 224 x 224. In contrast, automotive NPUs must run multiple, high-resolution networks concurrently, such as ResNext at 1920 x 1080 x 3, Swin Transformer at 2880 x 1860, and DETR 1824 x 940. Additionally, many OEMs have developed custom and proprietary NNs.
AI Compute Made for Automotive
Automotive applications demand new processing capabilities, including support for diverse neural networks, the ability to run multiple networks concurrently, extreme power efficiency, and high processor utilization—which avoids dark silicon waste.
Expedera addresses all these requirements. Our Origin™ E6 and E8 families can run multiple networks concurrently at up to 128 TOPS per single core and scale to PetaOPS with multiple cores. Furthermore, at an industry-leading 18 TOPS/W, Expedera requires no active cooling while meeting in-cabin and ADAS needs. With support for standard and custom NNs, including unknown future NNs, Expedera is the ideal IP partner for automotive chip design.
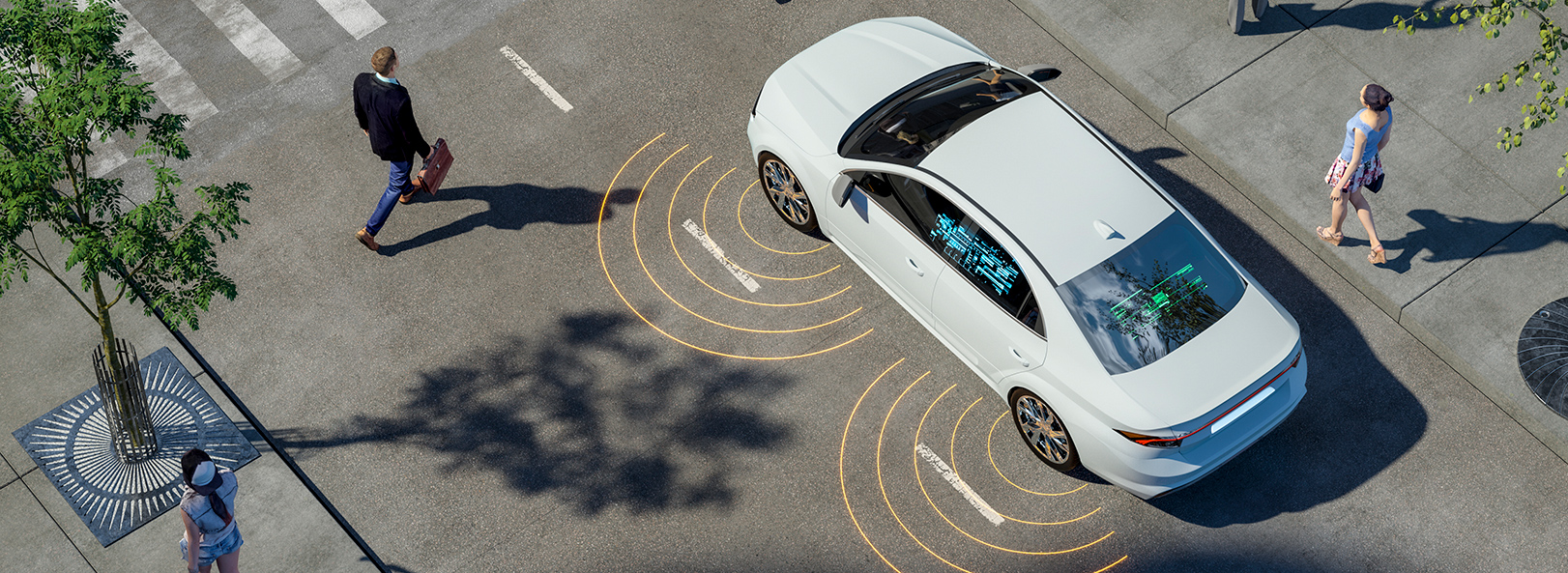
An Ideal Architecture for Automotive
The ASIL-B readiness certified Origin E8 neural engine uses Expedera’s unique packet-based architecture, which is far more efficient than common layer-based architectures. The architecture enables parallel execution across multiple layers, achieving better resource utilization and deterministic performance. It also eliminates the need for hardware-specific optimizations, allowing customers to run their trained neural networks unchanged without reducing model accuracy. This innovative approach greatly increases performance while lowering power, area, and latency.
An NPU that Scales Across Applications
From in-cabin to ADAS Level 3, L4, and L5, automotive AI performance requirements range from a few TOPS to 100s TOPS. Origin IP scales from GOPS to 128 TOPS in a single core. The architecture eliminates the memory sharing, security, and area penalty issues faced by lower-performing, tiled AI accelerator engines.
Choose the Networks You Need
New AI networks are rapidly emerging. Whether traditional CNN and RNN, transformer, LLM, grid sample, stable diffusion, or something new or proprietary, Expedera’s flexible architecture can address current and future network needs with highly optimized, power- and cost-sensitive NPU solutions.
Optimized for Model Accuracy
Expedera's packet-based architecture eliminates the need for hardware-specific optimizations, allowing customers to run their trained neural networks unchanged without reducing model accuracy. Origin IP and software stack support trained standard, custom, and proprietary neural networks as-is, saving customers the time and effort generally needed for hardware or software optimizations.
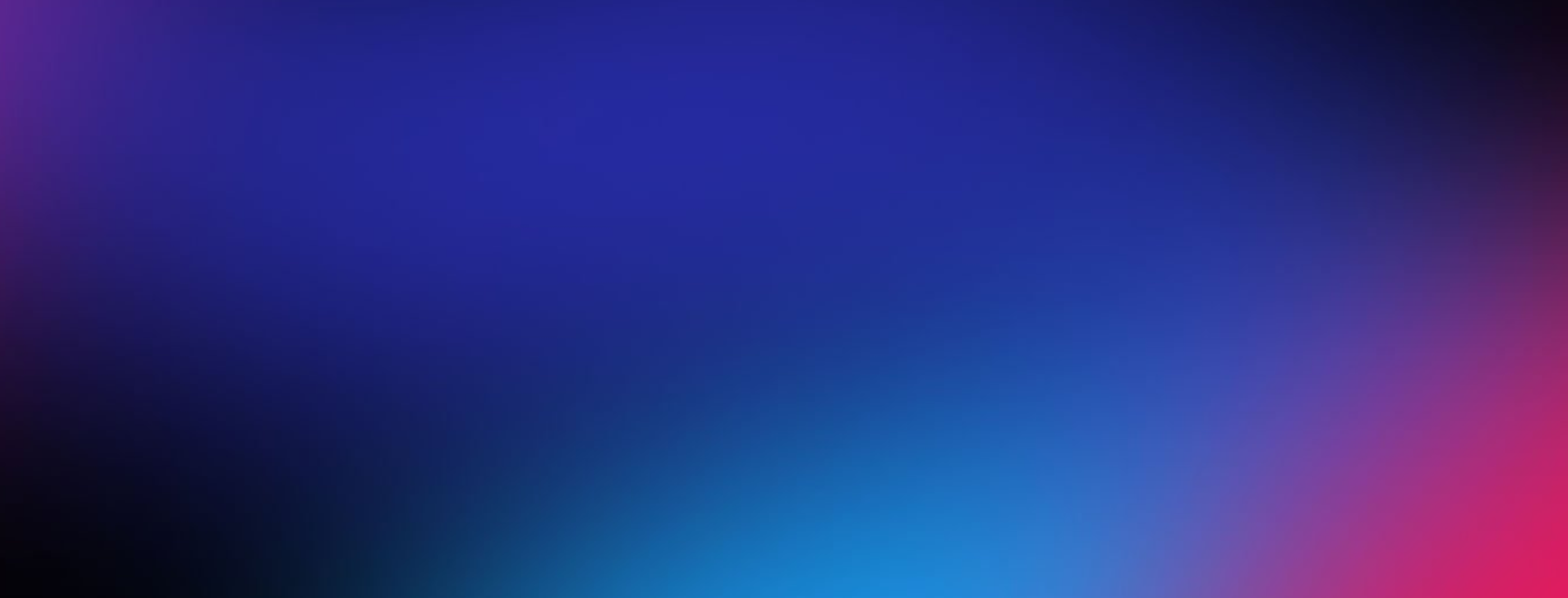
Download our White Papers
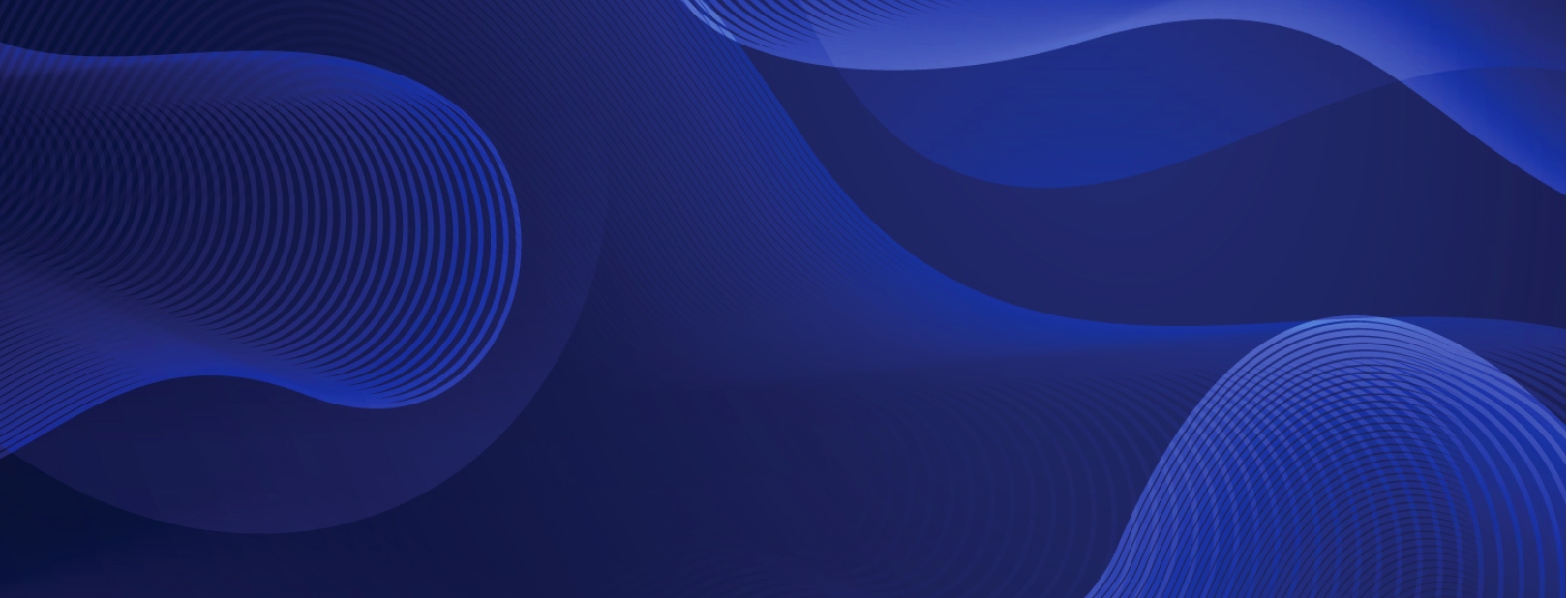